Two-Factor Authentication (sometimes referred to as 2FA) is a more secure method of logging into websites or online services.
Traditionally, when you “log in” to a website or online service, you enter your username (typically your email address) and password. Then you click a button, and if the details you enter are valid, you’re logged in.
Unfortunately, many people reuse the same credentials (username / password combination) again and again for multiple websites and online services. The danger of this is that if one of those services gets “hacked” or suffers a data breach where user credentials are exposed, an attacker could potentially then access all other websites and online services that that person uses.
Two-factor authentication combats this. It does so by employing a secondary means of authentication in addition to the traditional username / password combination in order to authenticate a user’s access.
This means that even if a user’s password has been compromised, an attacker couldn’t then this to gain access to someone’s account.
Two Factor Authentication in MIDAS
Since 2015, all MIDAS room booking systems have included optional two-factor authentication. If enabled, this adds an additional layer of account security to our software.
With Two-Factor Authentication enabled, each time a user logins in, a code is sent to their email inbox. The user must then enter this code into MIDAS in order to complete their log in.
But starting with MIDAS v4.38, we’re improving 2FA options and support in our software!
MIDAS v4.38 (and later) now support authenticator apps – including Google Authentication and Microsoft Authenticator – as an alternative 2FA login option to email.
Per User Two Factor Authentication Settings
Previously, the 2FA option in MIDAS was a ‘global’ setting. This meant that it could be enabled or disabled for all user accounts at once. It was not possible to have ‘per account’ 2FA settings.
We’ve made this more flexible for MIDAS v4.38!
Now, administrators can set whether 2FA is enabled for each individual user account. The 2FA option for each account can also be set.
Available 2FA options are now:
- Authenticator App
Enabling 2FA Authenticator App Globally in MIDAS
To globally turn on 2FA for all users, administrators can go to MIDAS Admin Options > Manage MIDAS > Security. In the “Two Factor Authentication (2FA)” section, tick the “Enable Two-Factor Authentication For All Users?” box, and then select the “Authenticator App” option:
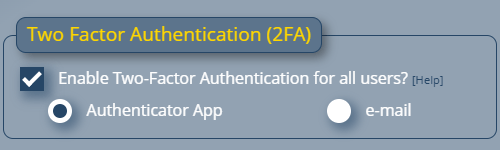
Click “Save Changes” and 2FA via Authenticator Apps will be enabled for all user accounts.
Enabling 2FA Authenticator App For Individual User Accounts
2FA options are also available on a per-user account basis. Administrators can enable, disable, or change the 2FA method on a user account by going to MIDAS Admin Options > Manage Users & Permissions.
Select the user account you wish to enable 2FA for, and choose “Authenticator App” from the “2FA Login” setting:
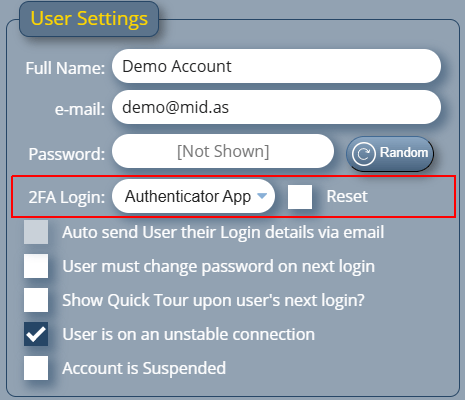
Then click “Save Changes”.
How 2FA via an Authenticator App Works
When 2FA authentication via authenticator apps has been enabled on a user’s account, the next time they login, they’ll be presented with a QR Code to scan with their authenticator app:
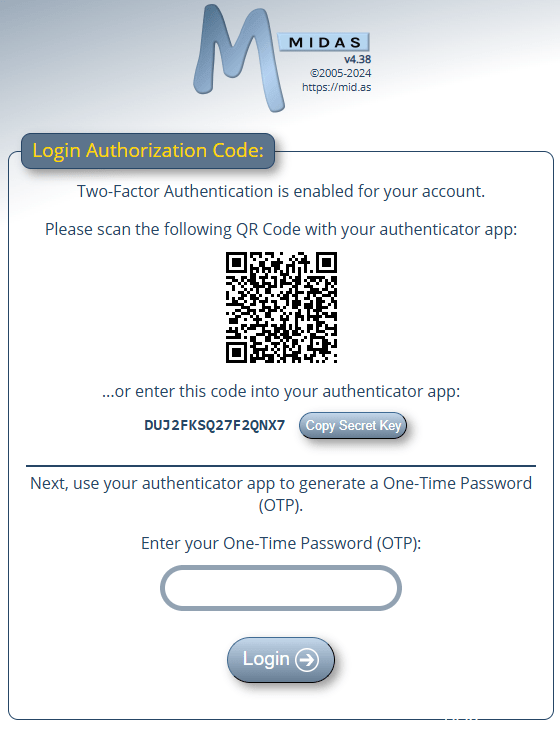
If they’re unable to scan the QR Code a ‘secret key’ is also provided which can be manually entered into authenticator apps.
The user’s authenticator app will then generate a 6 digit code which they can enter into MIDAS to complete their login.
The QR Code / Secret Key needs only to be scanned/entered into the user’s authenticator app once upon first use. For subsequent logins, the user will simply need to enter the 6 digit code generated by their authenticator app:
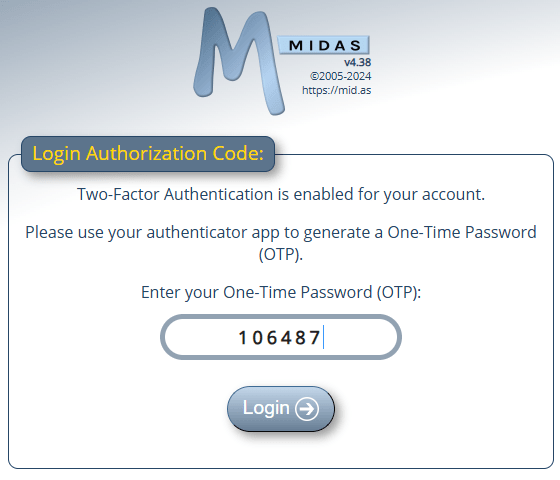
Supported Authenticator Apps
Popular FREE authenticator apps supported by MIDAS include:
- Google Authenticator (available for Android and iOS)
- Microsoft Authenticator (available for Android and iOS)
- Apple Passwords App (included starting with iOS 18 and macOS Sequoia)
- 2FAGuard (available for Windows)
- 2fast (available for Windows)
- Authme (available for Linux, macOS, and Windows)
- FirstOrder Authenticator (available for Android, iOS, macOS, and Windows)
- KeePassXC (available for MacOS, Windows, and Linux)
- Password Manager SafeInCloud (available for Android, iOS, macOS, and Windows)
- Protecc (available for Windows)
- SafeNet MobilePASS+ (available for Android, iOS, and Windows)
However, any OTP authenticator app which generates Timed One-Time Passwords (TOTP) derived from a shared secret value and the current time should be compatible. TOTP codes are typically six digits long and change every 30 seconds.
Resetting 2FA
If a user looses their authenticator app, an administrative user in a MIDAS system can change the user’s 2FA method, or reset their authenticator token. By resetting a user’s authenticator token, the next time the user logs in, they’ll be presented with a brand new QR Code/Secret Key to enter into their authenticator app.
Availability
2FA login authentication has been available since MIDAS v4.10 (2015). However, this implementation is limited to authentication codes sent to users via email. 2FA could also only be enabled globally (for all user accounts)
2FA login authentication via either email or authenticator apps is available in MIDAS v4.38 or later. These options can be enabled globally, or an a per user account basis.